Oh, look who’s back with their credit card in hand, ready to drop some serious dough on the latest GPU for their deep learning experiments. I get it – you want the biggest, worst graphics card to flex on your AI peers.
But hold up turbo. Sure, slapping in an RTX 4090 will make your neural networks purr, but that beast will also leave your wallet whimpering. Before you take out a second mortgage or sell your firstborn, let’s chat about intelligent options. See, you don’t need to break the bank for respectable performance.
The key is understanding your workload and real requirements. We’ll walk through the critical specs, decode the marketing hype, and highlight smart buys. You may not end up with the shiniest new toy, but your models will still churn and you’ll be able to pay your electricity bill. So plug in and let’s optimize your next GPU purchase.
Our Top Pick : Best GPU for AI & Machine Learning
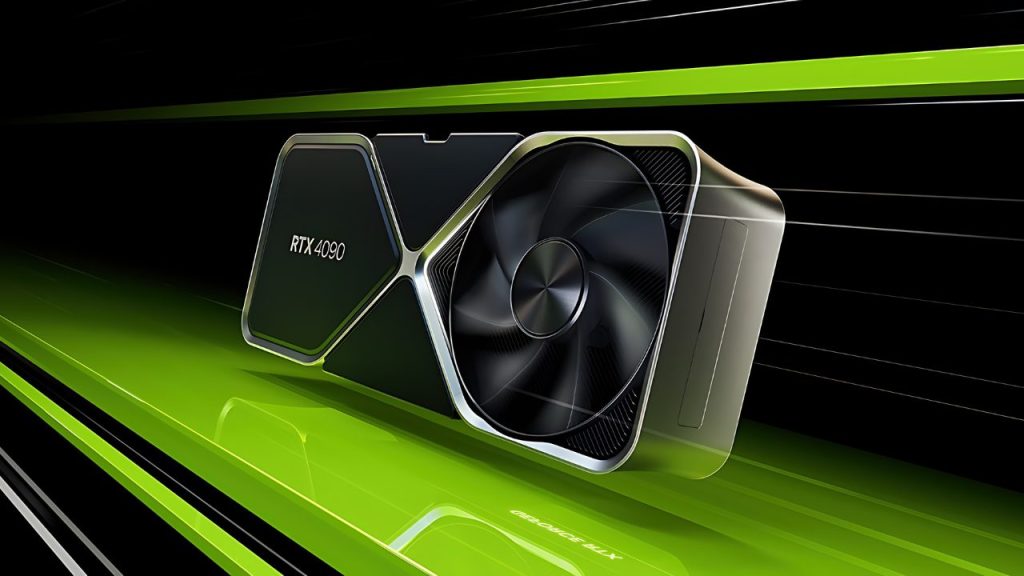
The Nvidia GeForce RTX 4090 graphics card is considered a good choice for AI tasks due to its ability to handle complex workloads and large datasets. It includes DLSS AI upscaling, which can improve the performance of deep learning models by up to 200%. This makes the RTX 4090 a good choice for both training and serving models.
GPU/Model | ResNet50 | SSD | Bert Base Finetune | TransformerXL Large | Tacotron2 | NCF |
GeForce RTX 3090 TF32 | 144 | 513 | 85 | 12101 | 25350 | 14714953 |
GeForce RTX 3090 FP16 | 236 | 905 | 172 | 22863 | 25018 | 25118176 |
GeForce RTX 4090 TF32 | 224 | 721 | 137 | 22750 | 32910 | 17476573 |
GeForce RTX 4090 FP16 | 379 | 1301 | 297 | 40427 | 32661 | 32192491 |
Read More : NPUs: Super-Brains That Make Your Laptop and Phone Smartest
Pros:
- High frame rates The RTX 4090 can consistently reach over 100 frames per second (fps) at 4K resolution and the highest graphics preset in most games.
- Real-time preview The RTX 4090 allows for real-time previews of work, which can be useful when working in programs like Blender.
Cons:
- Expensive The RTX 4090 has a high price tag of $1,600, which may be too much for some gamers.
- Power usage The RTX 4090 uses over 400 watts of power, which may be more than some other cards.
GPU Architecture for Deep Learning
So you want to do deep learning, huh? Well then, you’re going to need a graphics card that can handle the immense number crunching required. But don’t just grab the first GPU you see and call it a day. There are a few things you should consider to find one that fits your needs without emptying your wallet.
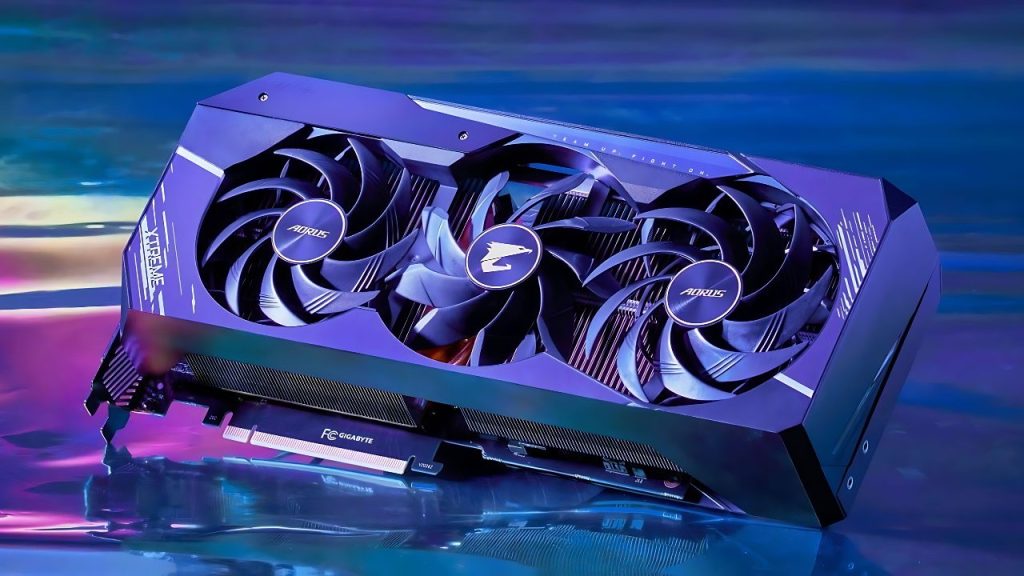
First, forget about VRAM. While video memory was important for gaming graphics cards of yore, it means diddly squat for deep learning. What really matters are the number of CUDA cores, which do the actual computing. The more cores the merrier, as they’ll get those neural networks trained in a hurry.
Speaking of cores, pay attention to Tensor Cores, NVIDIA’s specialized cores for deep learning matrix math. Tensor Cores provide a massive speed boost for deep learning, so try to find a card with at least 100 of these bad boys. The latest RTX 40 series from NVIDIA packs over 500 Tensor Cores, but those cards will also set you back a pretty penny.
Caches are also key. L1 and L2 caches store data right on the GPU so it can be accessed quickly. Bigger caches mean less waiting around for data and faster training times. The RTX 4090 has a whopping 144MB of cache, over 4X more than the previous generation.
In the end, you’ll have to weigh performance versus cost for your needs. If you’re just getting started with deep learning or building your first model, an older generation card with at least 100 Tensor Cores should work great without crushing your budget.
But if you’re doing intensive research or building massive models, you’ll want the latest and greatest GPU tech, cost be damned. The choice is yours, but choose wisely! Your GPU will determine how fast you can build and iterate on those neural networks.
NVIDIA Ampere RTX Series: Key Specs and Features
So, you’re in the market for a new graphics card and have your eye on NVIDIA’s latest RTX 40 Ampere series. Wise choice, my friend. These next-gen GPUs are poised to blow their Turing predecessors out of the water. But with great power comes…well, you know the rest. Picking the right RTX 40 Ampere card for your needs can be trickier than getting out of bed in the morning.
Cores Galore
The RTX 40 series is chock full of Tensor Cores, which are specialized cores used for deep learning operations. The more Tensor Cores, the faster your neural network training will be. The flagship RTX 5090 comes with a whopping 10496 Tensor Cores—over 5 times more than the RTX 3090. Even the RTX 4070 has 6144 Tensor Cores, so any RTX 40 card should handle deep learning tasks with aplomb.
Memory Matters
GPU memory, or VRAM, determines how large of a neural network you can train. More VRAM means you can work with bigger datasets and more complex models. The RTX 40 series offers from 16 GB up to a staggering 80 GB of GDDR6X memory. While the RTX 4090’s 80 GB of VRAM is overkill for most, even the RTX 4070’s 16 GB will allow you to train some hefty networks.
Performance Per Dollar
Of course, you’ll want the most bang for your buck. In terms of raw performance, the RTX 4090 is unparalleled but will set you back a cool $2,999. The mid-range RTX 4080 offers nearly as much power for $1,199, giving far better performance per dollar.
And the $599 RTX 4070 still provides a sizable boost over the last-gen RTX 3070 at a lower cost. So weigh your options carefully based on your needs and budget.
Any RTX 40 series card will give your deep learning pursuits a boost, but some may be overpowered (and overpriced) for your needs. My advice? Go for the RTX 4080—with its combination of cores, memory, and reasonable cost, it hits the sweet spot for most machine-learning maestros. But you do you!
Best GPUs for Deep Learning in 2024
If you want to delve into the futuristic world of deep learning in 2024, you’re going to need some serious graphics processing power.
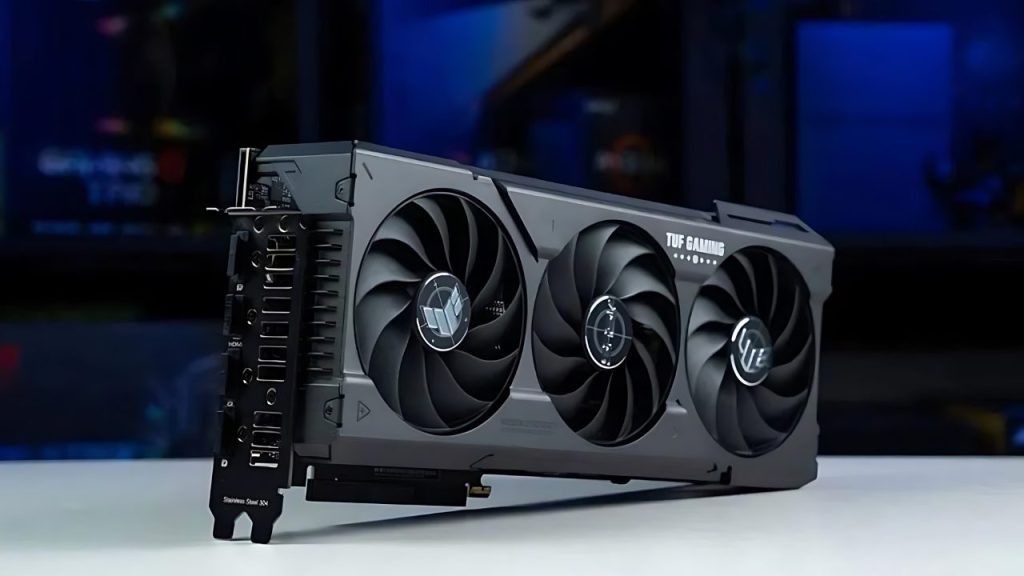
We’re talking about teraflops of computing muscle and gobs of graphics memory to handle massive datasets and complex algorithms. But with so many options on the market in a few years, how’s a budding data scientist to choose?
Read More : Choose a Laptop for Programming : A Comprehensive Guide 2024
Don’t Skimp on Memory
You’ll want at least 16 gigabytes of graphics memory, preferably more. Those neural networks have a lot of parameters to keep track of, ya know. Anything less and your GPU will choke on the bigger models, slowing your experiments to a crawl. While more memory may cost you, it’ll save you headaches (and wasted time) down the road.
Core Counts Matter
Not in the way you think, though. Forget comparing core counts across GPUs. Thanks to NVIDIA’s latest Ampere architecture, the number of cores alone won’t tell you how fast a GPU really is.
The Ampere Tensor Cores are where the real magic happens, providing huge boosts in throughput for deep learning workloads. So a GPU with fewer cores but more Tensor Cores may actually outperform one with more standard cores. The moral of the story? Don’t get too caught up in core counts.
Consider Your Cooling
Those powerhouse GPUs generate a lot of heat, and cooling is key to unlocking their full potential. If you’re building your own deep learning rig, invest in high-quality fans, heat sinks, and possibly even liquid cooling.
Laptop users should consider an external GPU enclosure that can properly dissipate the heat. Throttling your GPU due to overheating will only lead to frustration and subpar performance.
In the end, you want a GPU with plenty of fast memory, Tensor Cores optimized for deep learning, and enough cooling to handle the demands of your experiments. Following these tips will ensure you have a GPU that’s primed and ready to push the boundaries of AI in 2024 and beyond. The future is yours to create!
Best Budget GPUs for AI and Machine Learning
If you’re on a budget but still want to dip your toe into the exciting world of AI, you’ve got options. You don’t need the latest and greatest (read: most expensive) GPU with more cores than you have brain cells to get started. Any of the following picks will handle basic machine-learning tasks without exploding your wallet.
NVIDIA GTX 1660 Super
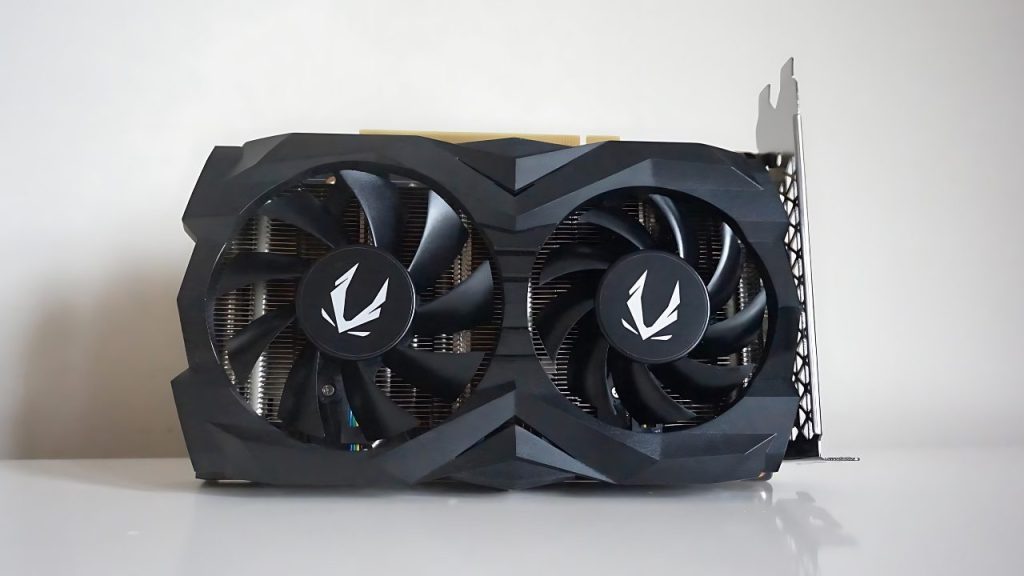
This plucky little GPU from NVIDIA packs a surprising punch for its size. With 6GB of VRAM and 1408 CUDA cores, the 1660 Super can handle common ML frameworks like TensorFlow and PyTorch.
It won’t set any speed records, but for under $250, it’s hard to beat for learning the basics. The 1660 Super is a solid starter GPU that won’t have you weeping when the power bill comes.
AMD Radeon RX 5700 XT
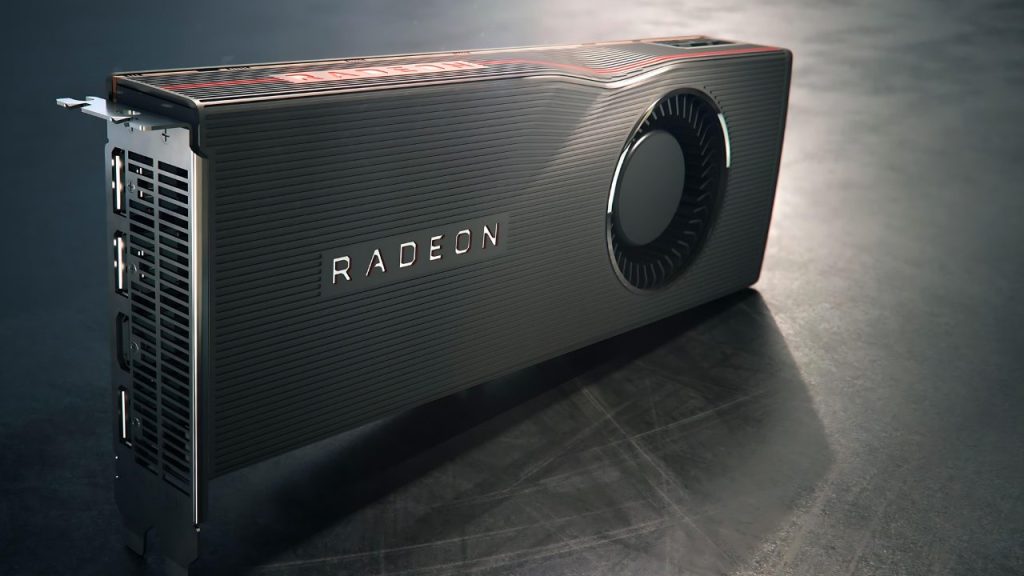
If you want to support the underdog, AMD’s RX 5700 XT is a compelling choice. For around $400, you get 8GB of VRAM and 2560 stream processors. The 5700 XT handles ML tasks reasonably well and has better raw performance than NVIDIA’s similarly-priced RTX 2060.
Just be aware that some ML software and frameworks are optimized for NVIDIA’s CUDA architecture, so you may encounter compatibility issues. But if you want to stick it to NVIDIA’s near-monopoly, the 5700 XT is a great way to do it on a budget.
NVIDIA RTX 2070 Super
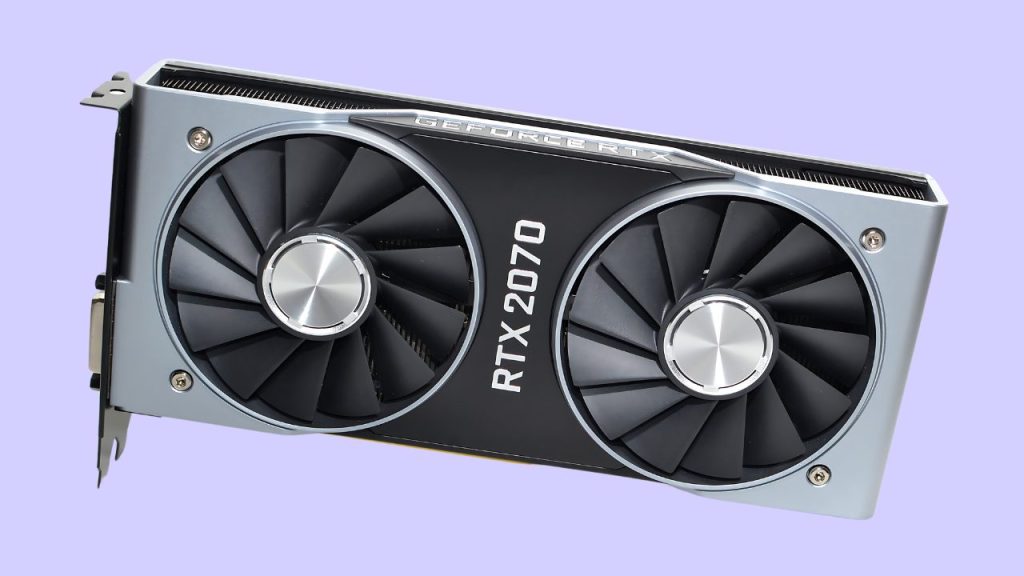
The RTX 2070 Super is a solid mid-range offering from NVIDIA for machine learning on a budget. For under $500, you get 8GB of fast GDDR6 memory and 2560 CUDA cores. The 2070 Super can handle more complex ML tasks than the other options on this list, with respectable performance for natural language processing, computer vision, and some deep learning models.
If you want to do more than just dip your toe in the ML waters without paying through the nose, the 2070 Super deserves a look. In the end, the best budget GPU for you depends on how serious you want to get with machine learning. But with options like these, you can start your AI adventures without paying an arm and a leg. Go forth and learn, my budget-conscious friends!
Conclusion
So there you have it, dear reader. Now that you’re armed with all this juicy knowledge about GPU guts and glory, you’ll be ready to stride into that electronics store like a boss and demand only the finest silicon for your deep learning needs.
Just remember – don’t let those salespeople try to upsell you with flashy lights and promises of marginally better performance. You know what you need, and you know the hard numbers. Stay strong, stay focused, and before you know it, you’ll be training models faster than a Tesla on autopilot. The future is yours for the taking. Go get it, tiger.
Read More : AMD vs. Intel: Which CPUs Are Better for Gaming PC in 2024